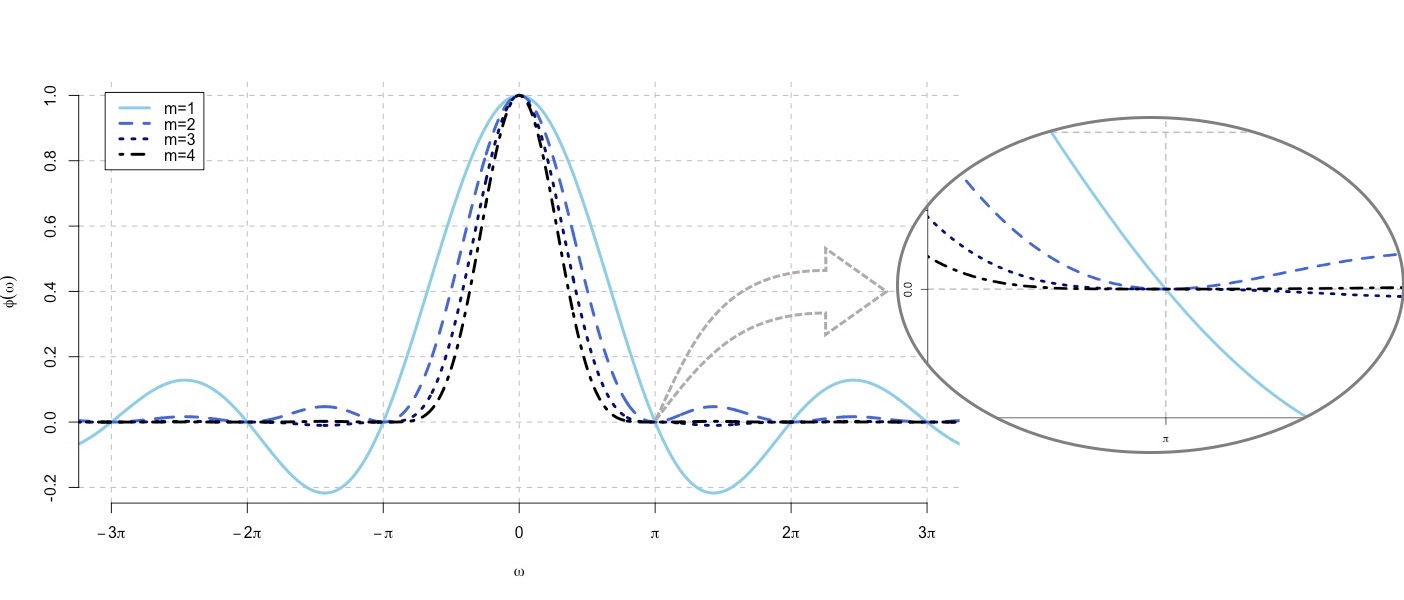
It is a conventional assumption in density deconvolution problems such that the characteristic function of the measurement error distribution is non-zeros on the real line. However, there are many instances of problems in which the assumption is violated, for example, with the convolution of uniform distribution in the figure. Goldenshluger and Kim (2021) investigate the problem with the non-standard error distributions -- namely, that their characteristic functions have zeros on the real line -- by ascertaining how the multiplicity of the zeros affects the estimation accuracy. For this investigation, we develop optimal minimax estimators and derive the corresponding lower bounds. From that result, the best achievable estimation accuracy is determined through the multiplicity of zeros, the rate of decay of the error characteristic function, and the smoothness and tail behavior of the estimated density. In addition, we consider the problem of adaptive estimation by proposing a data-driven estimator that automatically adapts to the unknown smoothness and tail behavior of the density to be estimated.